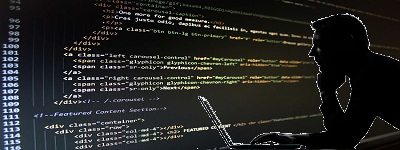
IntelのNCSDK Documentationからの翻訳です。
TensorFlowサポート
(TensorFlow Support)
TensorFlowネットワークのコンパイルのための手引き
(Guidance for Compiling TensorFlow Networks)
TensorFlow-Slimネットワークのコンパイルのための手引き
(Guidance for Compiling TensorFlow-Slim Networks)
TensorFlow Model Zooネットワークのコンパイルの手引き
(Guidance for Compiling TensorFlow™ Model Zoo Networks)
Caffeサポート
Caffeは、Berkeley AI Research(BAIR)とコミュニティの貢献者によって開発されたDeep Learningフレームワークです。
各バージョンのIntel Movidius Neural Compute SDK(Intel Movidius NCSDK)はそのリリースに幅広いネットワークサポートを提供するCaffeの単一バージョンをインストールし、検証されます。
インストールされている特定のバージョンは、リリースごとに変更される場合があります。
他のバージョンのCaffeはNCSDKで動作する可能性がありますが、正式にはサポートされておらず、特定の開発マシンのカスタマイズが必要な場合があります。
セットアップスクリプトは現在、SSD Caffeをダウンロードし、システムの場所にインストールします。
Caffeインストールへのソフトリンクは、/opt/movidius/caffeにあります。
Caffe Models
Caffeモデルは、NCSDK mvNCCompileツールを使用してcaffeモデルをコンパイルするために使用される
2つのファイルで構成されています。
.prototxt – ネットワークのトポロジとレイヤーを記述するテキストファイル
.caffemodel – モデルをトレーニングした後に得られる各レイヤーの重みを含むバイナリファイル
Note: Further reading: Deploying Your Customized Caffe Models on Intel® Movidius™ Neural Compute Stick
Caffe Layerのサポート
次のレイヤーはNCSDKによるCaffeでサポートされています。
NCSDKはネットワークトレーニングをサポートしていないため、
トレーニングにのみ必要なレイヤはサポートされていません。
Activation/Neuron
bias
elu
prelu
relu
scale
sigmoid
tanh
Common
inner_product
Normalization
batch_norm
lrn
Utility
concat
eltwise
flatten
parameter
reshape
slice
softmax
Vision
conv
Regular Convolution – 1x1s1, 3x3s1, 5x5s1, 7x7s1, 7x7s2, 7x7s4
Group Convolution – <1024 groups total
deconv
pooling
既知の問題点
Caffe Input Layer
制限:最初の次元であるバッチサイズは常に1でなければなりません
制限:入力の数は1でなければなりません
制限:入力層のこの “input_param”形式はサポートしていません。
name: “GoogleNet”
layer {
name: “data”
type: “Input”
top: “data”
input_param { shape: { dim: *10* dim: 3 dim: 224 dim: 224 } }
}
私たちは入力層のこの “input_shape”フォーマットのみをサポートしています:
name: “GoogleNet”
input: “data”
input_shape
{ dim:1 dim:3 dim:224 dim:224 }
Input Name
inputは常に”data”です。
これは動作します:
name: “GoogleNet”
input: “data”
input_shape
{ dim:1 dim:3 dim:224 dim:224 }
これは動作しません:
name: “GoogleNet”
input: “data_x”
input_shape
{ dim:1 dim:3 dim:224 dim:224 }
Crop Layer
制限: Crop layer cannot take reference size layer from input:”data”.
layer {
name: “score”
type: “Crop”
bottom: “upscore”
bottom: “data”
top: “score”
crop_param {
axis: 2
offset: 18
}
}
サイズ制限
Compiled Movidius™ “graph” file < 320 MB; Intermediate layer buffer size < 100 MB
[Error 35] Setup Error: Not enough resources on Myriad to process this network
Scratch Memory size < 112 KB
[Error 25] Myriad Error: “Matmul scratch memory [112640] lower than required [165392]”
TensorFlowサポート
TensorFlow *は、Googleが開発したDeep Learningフレームワークです。
インテル Movidius ニューラルコンピューティングSDK(インテル Movidius NCSDK)は、NCSDK v1.09.xxリリースでTensorFlowサポートを導入しました。
各リリースのTensorFlow検証は、リリースノートに記載されているTensorFlowバージョンで行われます。
デフォルトのインストール場所:/opt/movidius/tensorflow
TensorFlow Model Zoo
TensorFlowには、https://github.com/tensorflow/modelsのモデル用のGitHubリポジトリがあります。
それぞれの著者によって管理されているいくつかのモデルが含まれています。
TensorFlowネットワークのコンパイル
●NCSDKおよびNeural Compute APIで使用するためにTensorFlow Model Zooからモデルをコンパイルする場合は、
TensorFlow Model Zooネットワークのコンパイルの手引きに従ってください。
●NCSDKおよびNeural Compute APIで使用するTensorFlow-Slimネットワークをコンパイルする場合は、
TensorFlow-Slimネットワークのコンパイルのための手引きに従ってください。
●NCSDKおよびNeural Compute APIで使用するために独自のTensorFlowネットワークモデルをトレーニングする必要がある場合は、
TensorFlowネットワークのコンパイルのための手引きに従ってください。
サポートされるネットワーク
Inception v1
Inception v2
Inception v3
Inception v4
Inception ResNet v2
MobileNet_v1_1.0 variants:
MobileNet_v1_1.0_224
MobileNet_v1_1.0_192
MobileNet_v1_1.0_160
MobileNet_v1_1.0_128
MobileNet_v1_0.75_224
MobileNet_v1_0.75_192
MobileNet_v1_0.75_160
MobileNet_v1_0.75_128
MobileNet_v1_0.5_224
MobileNet_v1_0.5_192
MobileNet_v1_0.5_160
MobileNet_v1_0.5_128
MobileNet_v1_0.25_224
MobileNet_v1_0.25_192
MobileNet_v1_0.25_160
MobileNet_v1_0.25_128
特定のリリースでサポートされているネットワークのリリースノートを参照してください。
TensorFlowネットワークのコンパイルのための手引き
以下は、推論ではなく訓練のために構築されたTensorFlow *ネットワークのコンパイルに関する一般的なガイダンスです。
一般的なガイダンスは、TensorFlow GitHubリポジトリから利用できるmnist_deep.py への変更を示しています。
変更は典型的なdiff出力として表示され、行の先頭にある ‘ – ‘は行が削除されたことを示し、行の先頭にある ‘+’は
行が追加されることを示します。
‘ – ‘または ‘+’のない行は変更されておらず、文脈のために提供されています。
Neural Compute APIで使用するためにTensorFlowネットワークをコンパイルするには、展開/推論に固有のネットワークの
バージョンを保存し、トレーニング機能を省略する必要があります。
次の手順には、一般的なTensorFlow™ネットワークをコンパイルするためにユーザーが何を行う必要があるかが含まれています。
すべての手順がすべてのネットワークに適用されるわけではありませんが、一般的なガイダンスとして扱われるべきです。
●
ネットワークの最初のレイヤーに名前が設定されていることを確認してください。
これは厳密には必須ではありませんが、最初と最後のレイヤーに明示的に名前を付けていない場合は、
それらのレイヤーが与えられた名前を判別し、それらをコンパイラーに提供する必要があるためです。
mnist_deep.pyの場合、最初のノードに “input”という名前を付けるために次のように変更します。
1 2 3 |
# Create the model - x = tf.placeholder(tf.float32, [None, 784]) + x = tf.placeholder(tf.float32, [None, 784], <strong>name="input"</strong>) |
●
TensorFlowコードを追加して、訓練されたネットワークを保存します。 mnist_deep.pyについては、
訓練を受けたネットワークを保存するための変更は次のとおりです。
1 2 3 4 5 6 7 8 9 10 |
+ saver = tf.train.Saver() + with tf.Session() as sess: ... print('test accuracy %g' % accuracy.eval(feed_dict={ x: mnist.test.images, y_: mnist.test.labels, keep_prob: 1.0})) + + graph_location = "." + save_path = saver.save(sess, graph_location + "/mnist_model") |
●
コードを実行してネットワークをトレーニングし、訓練されたネットワークを保存するためにsaver.save()が
呼び出されていることを確認します。
プログラムが完了した後、成功した場合、saver.save()は次のファイルを作成します。
mnist_model.index
mnist_model.data-00000-of-00001
mnist_model.meta
●
ネットワークからトレーニング固有のコードを削除し、以前に保存したネットワークで読むためのコードを追加して、推論専用バージョンを作成します。
この手順では、元のTensorFlowコードを新しいファイルにコピーして新しいファイルを変更することをお勧めします。
たとえば、mnist_deep.pyを使って作業している場合、それをmnist_deep_inference.pyにコピーしておきます。
推論コードから削除するものは次のとおりです。
Dropout layers
Training specific code
Reading or importing training and testing data
Cross entropy/accuracy code
Placeholders except the input tensor.
ncsdkコンパイラは、未知のプレースホルダを解決しません。
多くの場合、余分なプレースホルダは特定の変数を訓練するために使用されるため、推論には必要ありません。
削除できないプレースホルダ変数は、推論グラフの定数に置き換えてください。
mnist_deep.pyの場合、以下の変更を行います
1 2 3 4 5 6 7 8 9 10 11 12 13 14 15 16 17 18 19 20 21 22 23 24 25 26 27 28 29 30 31 32 33 34 35 36 37 38 39 40 41 42 43 44 45 46 47 48 49 50 51 52 53 54 55 56 57 58 59 60 61 62 63 64 65 66 67 68 69 70 71 72 73 74 75 |
import tempfile - from tensorflow.examples.tutorials.mnist import input_data ... - # Dropout - controls the complexity of the model, prevents co-adaptation of - # features. - with tf.name_scope('dropout'): - keep_prob = tf.placeholder(tf.float32) - h_fc1_drop = tf.nn.dropout(h_fc1, keep_prob) ... - y_conv = tf.matmul(h_fc1_drop, W_fc2) + b_fc2 - return y_conv, keep_prob + y_conv = tf.matmul(h_fc1, W_fc2) + b_fc2 + return y_conv ... - # Import data - mnist = input_data.read_data_sets(FLAGS.data_dir, one_hot=True) ... - # Define loss and optimizer - y_ = tf.placeholder(tf.float32, [None, 10]) ... # Build the graph for the deep net - y_conv, keep_prob = deepnn(x) + # No longer need keep_prob since removing dropout layers. + y_conv = deepnn(x) ... - with tf.name_scope('loss'): - cross_entropy = tf.nn.softmax_cross_entropy_with_logits(labels=y_, - logits=y_conv) - cross_entropy = tf.reduce_mean(cross_entropy) - with tf.name_scope('adam_optimizer'): - train_step = tf.train.AdamOptimizer(1e-4).minimize(cross_entropy) - with tf.name_scope('accuracy'): - correct_prediction = tf.equal(tf.argmax(y_conv, 1), tf.argmax(y_, 1)) - correct_prediction = tf.cast(correct_prediction, tf.float32) - accuracy = tf.reduce_mean(correct_prediction) - graph_location = tempfile.mkdtemp() - print('Saving graph to: %s' % graph_location) - train_writer = tf.summary.FileWriter(graph_location) - train_writer.add_graph(tf.get_default_graph()) + + saver = tf.train.Saver(tf.global_variables()) + with tf.Session() as sess: sess.run(tf.global_variables_initializer()) + sess.run(tf.local_variables_initializer()) + # read the previously saved network. + saver.restore(sess, '.' + '/mnist_model') + # save the version of the network ready that can be compiled for NCS + saver.save(sess, '.' + '/mnist_inference') - for i in range(5000): - batch = mnist.train.next_batch(50) - if i % 100 == 0: - train_accuracy = accuracy.eval(feed_dict={ - x: batch[0], y_: batch[1], keep_prob: 1.0}) - print('step %d, training accuracy %g' % (i, train_accuracy)) - train_step.run(feed_dict={x: batch[0], y_: batch[1], keep_prob: 0.5}) - print('test accuracy %g' % accuracy.eval(feed_dict={ - x: mnist.test.images, y_: mnist.test.labels, keep_prob: 1.0})) - save_path = saver.save(sess, "./model.ckpt") |
●
最後のノードに名前が付いていることを確認します。
最初のノードと同様に、これは厳密には必須ではありませんが、コンパイルするには名前を知る必要があります。
これはノード名が “output”の最後のsoftmaxレイヤーを持つためにmnist_deep.pyに行う変更です:
1 2 3 4 |
# Build the graph for the deep net - y_conv, keep_prob = deepnn(x) + y_conv = deepnn(x) + output = tf.nn.softmax(y_conv, <strong>name='output'</strong>) |
●
ncsdkコンパイラを使用してコンパイルするのに適したセッションを保存するには、
コードの推論バージョンを実行します。
これは実際にネットワークを訓練していないので、NCSに優しい方法で再保存するだけで1秒しかかかりません。
実行後、成功すると、以下のファイルが作成されます。
mnist_inference.index
mnist_inference.data-00000-of-00001
mnist_inference.meta
●
次のコマンドで最終的に保存されたネットワークをコンパイルし、すべて動作する場合は、現在のディレクトリに
作成されたmnist_inference.graphファイルが表示されます。
コンパイルコマンドラインのTensorFlowネットワークの-wオプションの重み付けファイル接頭辞 “mnist_inference”
だけを渡すことに注意してください。
完全なコマンドは以下の通りです。
mvNCCompile mnist_inference.meta -s 12 -in input -on output -o mnist_inference.graph
TensorFlow-Slimネットワークのコンパイルのための手引き
Intel Movidius Neural Compute SDK(Intel Movidius NCSDK)およびNeural Compute APIで使用する
TensorFlow-Slimネットワークをコンパイルする場合は、以下の手順に従います。
下のコードは、TensorFlow セッションをグラフおよびチェックポイント情報とともに保存する方法を示しています。
import numpy as np
import tensorflow as tf
from tensorflow.contrib.slim.nets import inception
slim = tf.contrib.slim
def run(name, image_size, num_classes):
with tf.Graph().as_default():
image = tf.placeholder(“float”, [1, image_size, image_size, 3], name=”input”)
with slim.arg_scope(inception.inception_v1_arg_scope()):
logits, _ = inception.inception_v1(image, num_classes, is_training=False, spatial_squeeze=False)
probabilities = tf.nn.softmax(logits)
init_fn = slim.assign_from_checkpoint_fn(‘inception_v1.ckpt’, slim.get_model_variables(‘InceptionV1’))
with tf.Session() as sess:
init_fn(sess)
saver = tf.train.Saver(tf.global_variables())
saver.save(sess, “output/”+name)
run(‘inception-v1’, 224, 1001)
is_training = Falseパラメータは重要です。
これにより、トレーニング専用のレイヤー(NCSDKでサポートされていないレイヤー)はネットワークから除外されます。
次に、NCSDK mvNCCompileツールを使用して、NCSDKおよびNeural Compute APIで使用するために、保存したセッションを
上記のコードサンプルからコンパイルします。
mvNCCompile output/inception-v1.meta -in=input -on=InceptionV1/Logits/Predictions/Reshape_1 -s 12
TensorFlow Model Zooネットワークのコンパイルの手引き
TensorFlow Model Zooのモデルを、TensorFlowが提供するスクリプトを使用して、Intel Movidius Neural Compute SDK(Intel Movidius NCSDK)およびNeural Compute APIで使用するために簡単にコンパイルできます。
この図は、TensorFlowモデルをMovidius graphファイルに変換するプロセスの概要を示しています。
一般的な手順
TensorFlowソースコードとTensorFlowモデルリポジトリをクローン
git clone https://github.com/tensorflow/tensorflow.git
git clone https://github.com/tensorflow/models.git
チェックポイントファイルをダウンロードして抽出する
wget -nc http://download.tensorflow.org/models/<name of model tar file>.tar.gz
tar -xvf <name of model tar file>.tar.gz
GraphDefファイルをエクスポートする
python3 <path to TF models repo>/research/slim/export_inference_graph.py \
–alsologtostderr \
–model_name=<the name of the model> \
–batch_size=1 \
–dataset_name=<the name of the dataset> \
–image_size=<one dimension of image size> \
–output_file=<the name of the model>.pb
推論のためのFreeze Model
python3 <path to TF source repo>/tensorflow/python/tools/freeze_graph.py \
–input_graph=<the name of the model>.pb \
–input_binary=true \
–input_checkpoint=<the name of the model>.ckpt \
–output_graph=<the name of the model>_frozen.pb \
–output_node_name=<name of the output node>
Movidius graph ファイルをコンパイル
mvNCCompile -s <number of shaves> <name of the model>_frozen.pb -in=input -on=<name of the output node>
Inception v3モデルを使用した例
この例は、NCSDKで使用するInception v3モデルをコンパイルするための上記の手順を示しています。
チェックポイントファイルをダウンロードして抽出します
git clone https://github.com/tensorflow/tensorflow.git
git clone https://github.com/tensorflow/models.git
モデルのディレクトリを作成します
mkdir -p inception_v3
cd inception_v3
チェックポイントファイルをダウンロードして抽出します
wget -nc http://download.tensorflow.org/models/inception_v3_2016_08_28.tar.gz
tar -xvf inception_v3_2016_08_28.tar.gz
GraphDefファイルをエクスポートする
python3 ../models/research/slim/export_inference_graph.py \
–alsologtostderr \
–model_name=inception_v3 \
–batch_size=1 \
–dataset_name=imagenet \
–image_size=299 \
–output_file=inception_v3.pb
推論のためのFreeze Model
python3 ../tensorflow/tensorflow/python/tools/freeze_graph.py \
–input_graph=inception_v3.pb \
–input_binary=true \
–input_checkpoint=inception_v3.ckpt \
–output_graph=inception_v3_frozen.pb \
–output_node_name=InceptionV3/Predictions/Reshape_1
Movidius graph ファイルをコンパイル
mvNCCompile -s 12 inception_v3_frozen.pb -in=input -on=InceptionV3/Predictions/Reshape_1
graphバイナリの使われ方
では、バイナリはどんな風に使われているのかを参考までに。
これはYOLO.caffemodelでPythonコードの中の例ですが、他でもこんな具合です。
#graphバイナリファイル名を指定
network_blob=’graph’
……
# load blob
with open(network_blob, mode=’rb’) as f:
blob = f.read()
graph = device.AllocateGraph(blob)
graph.SetGraphOption(mvnc.GraphOption.ITERATIONS, 1)
iterations = graph.GetGraphOption(mvnc.GraphOption.ITERATIONS)
……
#load tensor
graph.LoadTensor(im.astype(np.float16), ‘user object’)
out, userobj = graph.GetResult()
….
#結果
# fc27 instead of fc12 for yolo_small
results = interpret_output(out.astype(np.float32), img.shape[1], img.shape[0])
….
#終了
graph.DeallocateGraph()
Leave a Reply