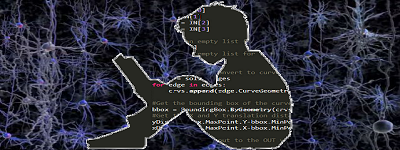
こういうサイトが公開されていました。
注:以下のリンクが本日(2019/07/25)あたりから切れています。昨日はアクセス過多で「Back Soon」なんていうメッセージになってました。復活まで待ちましょう。
写真をアップすると肖像画に変換されます。
が、眼目になるのはこれが「肖像画風に描画される」のではなく、「肖像画として描画される」ということです。
「HOW」を読むと、これはGAN(Generative Adversarial Network)を使ったAIプロジェクトだということなのですが、むしろ注目なのは「WHY」で書かれていることです。
この説明に沿うなら、もし我々が誰かの手による爆笑した、あるいは破顔一笑した様々なスタイルの45000枚の肖像画を用意できるなら、取り澄ました表情のあなたの写真から「大笑いしている肖像画」を作成できる…かも。でも、肖像画の歴史的経緯を尊重するなら、そんな肖像画….だれも欲しがらないでしょうってことかな?
つたない翻訳ですが、読む価値があるので掲載しておきます(特に最後の方)。
(一部、管理人には翻訳不可な1文がありました、そのままにしています)
In the early 1500s Lorenzo Lotto and Giovan Battista Moroni began the psychological analysis through portraiture. From this moment, the focus on the sitter’s identity becomes the leitmotif in the history of portrait. Portraits interpret the external beauty, social status, and then go beyond our body and face. A portrait becomes a psychological analysis and a deep reflection on our existence.
1500年代初頭、ロレンツォ・ロトとジョバン・バティスタ・モロニは肖像画を通して心理分析を始めました。 この瞬間から、座像のアイデンティティへの焦点は肖像画の歴史の中でライトモチーフになります。
肖像画は、外的な美しさ、社会的地位を解釈し、私たちの身体や顔を超えていきます。肖像画は心理分析となり、私たちの存在への深い考察となるのです。
AI Portraits Ars uses Artificial Intelligence to reproduce artistic human portraits, with different styles and levels of abstraction. For our model training, we adopt a data set of tens of thousands of paintings from the Early Renaissance to Contemporary Art. This type of portraiture is quite distinctive of the Western artistic tradition. Training our models on a data set with such strong bias leads us to reflect on the importance of AI fairness. In the previous work AI Portraits Celebrity, we explored the concept of micro-bias linked to the training data of only actors, which in some way imposes an actorization of the user’s portrait: “a collection of faces from the society of spectacle that are sedimented in the neural network, and vaporize my selfie in a cinematographic self.”
AI Portraits Ars introduces a very different type of bias with unique themes to explore.
AI Portraits Arsは人工知能を使用して、さまざまなスタイルと抽象化のレベルで芸術的な人間の肖像画を再現します。 モデルの訓練のために、私たちは初期ルネサンスから現代美術までの何万もの絵画のデータセットを採用しました。
この種の肖像は西洋の芸術的伝統とは非常に際立っています。 このように強いバイアスをかけてデータセットに基づいてモデルをトレーニングすると、AIの公平性の重要性について考えることができます。
以前の作品「AI Portraits Celebrity」では、俳優だけのトレーニングデータにリンクされたマイクロバイアスの概念を検討しました。これは、ある意味でユーザーの肖像を俳優化することを意味します。“a collection of faces from the society of spectacle that are sedimented in the neural network, and vaporize my selfie in a cinematographic self.”
AI Portraits Arsは探検するべき独特な主題との非常に異なったタイプのバイアスを紹介します。
Portraiture has a diverse and ancient history spanning geography and religion. It is discussed in the works of Aristotle, Plato, Cicero, and Pliny the Elder. We have incredible examples such as the Fayum portraits from Egypt. In other regions of Africa, there are the masks that are stylized to represent a character. In China, a portrait tradition can be traced back to the Han dynasty in 200 BC. In India, we have portrait miniature painting with the Mughal dynasty of the 17th century. In traditional Jewish and Islamic cultures, a prohibition on imagery made portraiture a taboo.
肖像画は地理学と宗教にまたがる多様で古くからの歴史を持っています。 それはアリストテレス、プラトン、シセロ、そしてプリニー・ザ・エルダーの作品で議論されています。 エジプトのFayum肖像画のような素晴らしい例があります。 アフリカの他の地域では、キャラクターを表すために様式化されたマスクがあります。 中国では、肖像画の伝統は紀元前200年の漢王朝にさかのぼることができます。 インドでは、17世紀のムガル王朝の肖像画のミニチュア絵画があります。 伝統的なユダヤ人やイスラム文化では、肖像画がタブー視されるようになったのは、描写の禁止があったからです。。
In AI Portraits Ars, we focus on the 15th century Europe, which is considered by art historians like Joanna Woodall, Shearer West, John Berger and many others, as a stylistic inflection point in the history of portraiture marked by the emergence of realistic depictions of individuals. Before the 15th century, the practice of commissioned painted portraits of individual sitters was rare. Perhaps this change in style reflects a shift in societal values toward individualism. The Italian Renaissance of the late 13th century, with the writings of Dante and the paintings of Giotto, was a period of increased self-consciousness, in which concepts of unique individual identity began to be verbalized. This was followed by the Renaissance glorification of the genius of woman and man, the representation of the unique and extraordinary ability of the human mind.
AI Portraits Arsでは、Joanna Woodall、Shearer West、John Bergerなどの美術史家によって,人物の現実的な描写の出現によって特徴付けられる肖像画の歴史の中で様式的な変曲点として考えられている15世紀ヨーロッパに焦点を当てています。
15世紀以前には、個々が座った絵の肖像画の習慣はまれでした。
おそらく、このスタイルの変化は、社会主義的価値観の個人主義へのシフトを反映しています。 13世紀後半のイタリアのルネサンスは、ダンテの著作とジョットの絵画と共に、自意識が高まり、独自の個性の概念が言葉で表現されるようになりました。
これに続いてルネサンスは、女性と男性の天賦の才、人間の心のユニークで並外れた能力を表現したものとして、その栄光を讃えました。
We encourage you to experiment with the tool as a way of exploring the bias of the model. For example, try smiling or laughing in your input image. What do you see? Does the model produce an image without a smile or laugh?
Portrait masters rarely paint smiling people because smiles and laughter were commonly associated with a more comic aspect of genre painting, and because the display of such an overt expression as smiling can seem to distort the face of the sitter. This inability of artificial intelligence to reproduce our smiles is teaching us something about the history of art.
(AI)モデルのバイアスを調べる方法として、このツールを試してみることをお勧めします。 たとえば、入力画像の中で笑ったり微笑んだりしてみてください。 何が見えますか? モデルは微笑みも笑いもなしにイメージを作り出しますか?
肖像画マスター(画家・巨匠)は笑顔の人々を描くことはめったにありません。微笑みと笑いはジャンル絵画のより漫画的な側面と一般的に関連していたし、そのようなあからさまな表情の笑顔の表示は、座っている人の顔をゆがめているように見えることがあるからです。
人工知能が私たちの笑顔を再現することができないことは、私たちに芸術の歴史について何かを教えています。
This and other biases that emerge in reproducing our photos with AI Portraits Ars are therefore an indirect exploration of the history of art and portraiture.
AI Portraits Arsで写真を複製する際に浮き彫りになるこのバイアスやその他のバイアスは、芸術と肖像の歴史を間接的に探究することです。
例えばこんな感じ。
「HOW」から抜粋
AI Portraits Ars is able to paint portraits in real time at 4k resolution. You will find yourself in front of a mirror and feel thousands Rembrandt, Caravaggio, Titian portraying you moment after moment.
AI Portraits Arsは4kの解像度でリアルタイムで肖像画を描くことができます。 あなたは自分自身を鏡の前で見つけ、何千人ものレンブラント、カラヴァッジョ、ティティアンがあなたを瞬間的に描いているのを感じるでしょう。
We have trained Generative Adversarial Network (GAN) models to reproduce human portraits, with different styles and levels of abstraction. GANs are a very popular class of deep generative models. They are trained to learn a mapping of a latent vector z ∈ Z to a generated image y = G(z) with G being the generator. The latent space Z describes all possible portraits. AI Portraits Ars pushes us towards an intuitive and playful way of interacting with state-of-the-art GAN models. By showing our face to the neural network, we walk through the Z space and identify the vector that best describes our face in the multidimensional space of the GAN. We trained AI Portraits Ars using our GAN on 45,000 portrait images. To allow insertion of own images into the latent space of a model, we trained an inverter that can approximate the latent vector z = I(x) from an image x.
我々は、異なったスタイルと抽象化のレベルで、人間の肖像画を再現するために敵対的生成ネットワーク(GAN)モデルを訓練しました。 GANは非常に人気のあるクラスの深層生成モデルです。 それらは、生成ベクトルをGとして、生成された画像y = G(z)への潜在ベクトルz∈Zのマッピングを学ぶように訓練されています。 潜在空間Zはすべての可能な肖像画を記述します。 AI Portraits Arsは、最先端のGANモデルと対話するための直感的で遊び心のある方法を私たちに推し進めています。 私たちの顔をニューラルネットワークに見せることによって、Z空間を歩き回り、GANの多次元空間で自分の顔を最もよく表すベクトルを特定します。 私たちは45,000のポートレート画像で私たちのGANを使ってAI Portraits Arsを訓練しました。 モデルの潜在空間に自身の画像を挿入できるようにするために、画像xから潜在ベクトルz = I(x)を近似できるインバータを学習しました。
The GAN is composed of two neural networks, one learns to recognize portraits of people (Discriminator), and the other learns to generate them (Generator).
GANは2つのニューラルネットワークで構成されています。1つは人々の肖像を認識することを学び(Discriminator)、もう1つはそれらを生成することを学びます(Generator)。
This is not a style transfer
With AI Portraits Ars anyone is able to use GAN models to generate a new painting, where facial lines are completely redesigned. The model decides for itself which style to use for the portrait. Details of the face and background contribute to direct the model towards a style.
In style transfer, there is usually a strong alteration of colors, but the features of the photo remain unchanged. AI Portraits Ars creates new forms, beyond altering the style of an existing photo.
これはスタイル転写ではありません
AI Portraits Arsを使えば、GANモデルを使って新しい絵を描くことができます。ここでは、顔の線が完全に再設計されています。 (GAN)モデルは自分自身に対してどのスタイルをポートレートに使用するかを決定します。 顔と背景の詳細は、 (GAN)モデルをスタイルに向けるのに役立ちます。
スタイルの転写では、通常色の強い変更がありますが、写真の機能は変わりません。 AI Portraits Arsは、既存の写真のスタイルを変更するだけでなく、新しいフォームを作成します。
このGANを使ったAIプロジェクトで使われているアルゴリズムが何をやっているのか興味が尽きないです。
いわゆる顔認識を含んではいるのでしょうが、認識した上で何をやっているんでしょうね?
右の絵は左の写真を見て機械が描きましたと言われて「ああ、そうなんですか。お上手ですね。」と簡単に納得するのは難しいです。
顔認識でやったように肖像画の顔画像をベクトル化しているのかなあと思う。
例えば、ここではヤットさんの顔画像を128次元のベクトルにエンコードしています。
45000枚から学習した肖像画のベクトルデータから近似した肖像画を探して、写真のお顔をその肖像画にマッチさせるようにモーフィングしているのかな?
でももしそうだとしても、そのモーフィングのプロセスが分からない。
まぁ、ノイズをいじってその結果を見て推測しないとGANの場合、何やってるのか分からないみたいだけど。
ところで、上の記述にあるinverterって何だろう?
要調査
参照
arXivの論文
Towards Open-Set Identity Preserving Face Synthesis
「顔画像からidentityとattributesを別々に再構成する、GANに基づいたOpen-Set Identity Generating Adversarial Networkの提案。 face synthesis networkは、ポーズや感情、照明、背景などをキャプチャする属性ベクトルを抽出することができる。図中の2つの入力画像AおよびBから抽出された識別を再結合することによって、A’およびB’を生成することができる。
顔の正面化、顔属性モーフィング、 face adversarial example detectionなど、より広範なアプリケーションに応用可能。」
だそうです。
もしAI PortraitsのURLが永久に閉じられても、部分的にこの論文から再構築できる…..かもしれんです。
「あなたもモナ・リザになろう!」…….とかなんとか。
GitHubには個人でチャレンジしている方もいらっしゃるようです(問題山積のようですが)。
ResearchGateでも盛んに議論さているようなので、こういうテーマはメジャーなのかな?
Leave a Reply