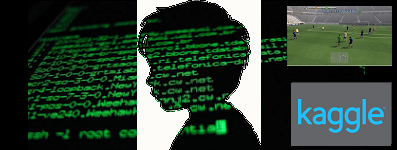
Kaggleでやる気になるために、ページを訳してみました。メモみたいなもんなので、変な訳でも気にしない(^^)。
実際やる場合は、Kaggleのアカウントを取得しておいてください。
Google Research Football with Manchester City F.C.
Description(説明)
Manchester City F.C. and Google Research are proud to present AI football competition using the Google Research Football Environment.
マンチェスターシティF.C. とGoogleResearchは、GoogleResearchフットボール環境を使用してAIフットボールコンペを開催できることを誇りに思っています。
A word from Manchester City F.C.(マンチェスターシティからひと言)
Brian Prestidge, Director of Data Insights & Decision Technology at City Football Group, the owners of Manchester City F.C., sets out the challenge. “Football is a tough environment to perform in and an even tougher environment to learn in. Learning is all about harnessing failure, but failure in football is seldom accepted. Working with Google Research’s physics based football environment provides us with a new place to learn through simulation and offers us the capabilities to test tactical concepts and refine principles so that they are strong enough for a coach to stake their career on.”
マンチェスターシティF.C.のオーナーであるCityFootballGroupのDataInsights&DecisionTechnologyのディレクターであるBrianPrestidgeが課題を提示します。 「フットボールというのは実行するのが難しい環境であり、学ぶのはさらに難しい環境です。学習とは失敗を利用することですが、フットボールの失敗が受け入れられることはめったにありません。 Google Researchの物理ベースのフットボール環境と連携することで、シミュレーションを通じて学ぶための新しい場所が提供され、戦術的な概念をテストして原則を洗練し、コーチがキャリアを積むのに十分な強さを得ることができます。
“We are therefore very pleased to be working with Google’s research team in creating this competition and are looking forward to the opportunity to support some of the most creative and successful competitors through funding and exclusive prizes. We hope to establish ongoing collaboration with the winners beyond this competition, and that it will provide us all with the platform to explore and establish fundamental principles of football tactics, thus improving our ability to perform and be successful on the pitch.”
「したがって、このコンペの作成にGoogleの研究チームと協力できることを非常に嬉しく思います。また、資金提供と特別賞を通じて、最も創造的で成功している他の競争相手をサポートする機会を楽しみにしています。 このコンペティションを超えて受賞者との継続的なコラボレーションを確立し、フットボール戦術の基本原則を探求して確立するためのプラットフォームを私たち全員に提供し、ピッチでのパフォーマンスと成功の能力を向上させたいと考えています。」
Greg Swimer, Chief Technology Officer at City Football Group added “Technologies such as Machine Learning and Artificial Intelligence have huge future potential to enhance the understanding and enjoyment of football for players, coaches and fans. We are delighted to be collaborating with Google’s research team to help broaden the knowledge, talent, and innovation working in this exciting and transformational area”.
シティフットボールグループの最高技術責任者であるグレッグスイマーは、次のように述べています。「機械学習や人工知能などのテクノロジーは、プレーヤー、コーチ、ファンのフットボールの理解と楽しみを高める大きな将来の可能性を秘めています。Googleの研究チームと協力できることをうれしく思います。 このエキサイティングで変革的な分野で働く知識、才能、革新を広げるのに役立ちます。」
The Google Research football environment competition(GoogleResearchのフットボール環境コンペ)
The world gets a kick out of football (soccer in the United States). As the most popular sport on the planet, millions of fans enjoy watching Sergio Agüero, Raheem Sterling, and Kevin de Bruyne on the field. Football video games are less lively, but still immensely popular, and we wonder if AI agents would be able to play those properly.
世界はフットボールから非常な喜びを感じます(米国ではサッカー)。 地球上で最も人気のあるスポーツとして、何百万人ものファンがフィールドでセルヒオ・アグエロ、ラヒーム・スターリング、ケビン・デブライネを見ることを楽しんでいます。 フットボールのビデオゲームはそれほど活気がありません、それでも非常に人気がありますが、AIエージェントがそれらを適切にプレイできるかどうかは疑問に思っています。
Researchers want to explore AI agents’ ability to play in complex settings like football. The sport requires a balance of short-term control, learned concepts such as passing, and high-level strategy, which can be difficult to teach agents. A current environment exists to train and test agents, but other solutions may offer better results.
研究者は、フットボールのような複雑な環境で遊ぶAIエージェントの能力を調査したいと考えています。 このスポーツには、短期間の制御、パスなどの学習された概念、およびエージェントに教えるのが難しい可能性のある高レベルの戦略のバランスが必要です。 エージェントをトレーニングおよびテストするため現在の環境が存在しますが、他のソリューションがより良い結果を提供する可能性があります。
The teams at Google Research aspire to make discoveries that impact everyone. Essential to their approach is sharing research and tools to fuel progress in the field. Together with Manchester City F.C., Google Research has put forth this competition to get help in reaching their goal.
Google Researchのチームは、すべての人に影響を与える発見を目指しています。 彼らのアプローチに不可欠なのは、この分野での進歩を促進するための研究とツールを共有することです。 Google Researchは、マンチェスターシティF.C.と協力して、目標を達成するための支援を得るためにこのコンペを開催しました。
(3Dでシミュレーション)
In this competition, you’ll create AI agents that can play football. Teams compete in “steps,” where agents react to a game state. Each agent in an 11 vs 11 game controls a single active player and takes actions to improve their team’s situation. As with a typical football game, you want your team to score more than the other side. You can optionally see your efforts rendered in a physics-based 3D football simulation.
このコンペでは、フットボールをすることができるAIエージェントを作成します。 チームは、エージェントがゲームの状態に反応する「ステップ(steps)」で競争します。 11対11のゲームの各エージェントは、1人のアクティブなプレーヤーを制御し、チームの状況を改善するためのアクションを実行します。 典型的なフットボールの試合と同様に、あなたはあなたのチームが対戦側よりも多く得点することを望みます。 オプションで、物理ベースの3Dフットボールシミュレーションでレンダリングされた作業を確認できます。
If controlling 11 football players with code sounds difficult, don’t be discouraged! You only need to control one player at a time (the one with the ball on offense, or the one closest to the ball on defense) and your code gets to pick from 1 of 19 possible actions. We have prepared a getting started example to show you how simple a basic strategy can be. Before implementing your own strategy, however, you might want to learn more about the Google Research football environment, especially observations provided to you by the environment and available actions. You can also play the game yourself on your computer locally to get better understanding of the environment’s dynamics and explore different scenarios.
コードで11人のフットボール選手をコントロールするのが難しいと思われる場合でも、がっかりしないでください。 一度に1人のプレーヤー(攻撃のボールを持っているプレーヤー、または防御のボールに最も近いプレーヤー)を制御するだけで、コードは19の可能なアクションの1つから選択できます。 基本的な戦略がいかに簡単であるかを示すために、入門例を用意しました。 ただし、独自の戦略を実装する前に、Google Researchのフットボール環境、特に環境によって提供される観測結果と利用可能なアクションについて詳しく知りたい場合があります。 また、ローカルのコンピューターでゲームを自分でプレイして、環境のダイナミクスをよりよく理解し、さまざまなシナリオを探索することもできます。
If successful, you’ll help researchers explore the ability of AI agents to play in complex settings. This could offer new insights into the strategies of the world’s most-watched sport. Additionally, this research could pave the way for a new generation of AI agents that can be trained to learn complex skills.
成功すれば、研究者が複雑な環境で遊ぶAIエージェントの能力を探求するのに役立ちます。 これは、世界で最も注目されているスポーツの戦略への新しい洞察を提供する可能性があります。 さらに、この研究は、複雑なスキルを習得するためのトレーニングが可能な新世代のAIエージェントへの道を開く可能性があります。
どうやって勝ち負けを評価するか書かれています・・・・・省略
コンペ参加のデッドラインなどが書かれています・・・・・省略
$6000をどうやって山分けするかや勝ったらどんなご褒美がもらえるか書かれています・・・・・省略
Google Football Rules(グーグルフットボールのルール)
Rules(ルール)
In this competition you control a single player in 11-player teams. Rules are similar to the official football (soccer) rules – https://www.rulesofsport.com/sports/football.html, including offsides, yellow and red cards. There are, however, small differences:
このコンペでは、11人のプレーヤーのチームで1人のプレーヤーをコントロールします。 ルールは公式のフットボール(サッカー)ルール – https://www.rulesofsport.com/sports/football.html に似ています-オフサイド、イエローカード、レッドカードを含みます。 ただし、小さな違いがあります。
●Game consists of two halves, 45 minutes (1500 steps) each. Kick-off at the beginning of each half is done by a different team, but there is no sides swap (game is fully symmetrical).
ゲームはそれぞれ45分(1500ステップ)ハーフで構成されます。 各ハーフの開始時のキックオフは異なるチームによって行われますが、サイドの入れ替えはありません(ゲームは完全に対称的です)。
●Each agent controls a single player on the team. Controlled player is always the one in the ball’s possession or the one close to the ball when defending.
各エージェントは、チームの1人のプレーヤーを制御します。 制御されたプレーヤーは、常にボールを持っているプレーヤー、または防御するときにボールに近いプレーヤーです。
●Teams do not switch sides during the game. Left/right sides are assigned randomly.
チームはゲーム中にサイドを切り替えません。 左側/右側はランダムに割り当てられます。
●For convenience of agent’s implementation, observations provided to the agent are always presented as if the agent controlled the left team. Environment applies appropriate conversions to both observations and actions. Game engine is fully symmetrical, so sides swapping does not affect the game in any way.
エージェントの実装の便宜のために、エージェントに提供される観測は、エージェントが左側のチームを制御しているかのように常に提示されます。 環境は、観測と行動の両方に適切な変換を適用します。 ゲームエンジンは完全に対称であるため、サイド入れ替えはゲームにまったく影響しません。
●Non-cup scoring rules apply, i.e. the team which scored more goal wins; otherwise it is a draw.
カップ戦のルールは適用されません。つまり、より多くの得点を挙げたチームが勝ちます。 それ以外の場合は引き分けです。
●There is no walkover applied when the number of players on the team goes below 7.
チームのプレーヤー数が7を下回った場合、ウォークオーバーは適用されません(つまり、不戦勝というのはありませんーレッドカードルールがあるんで、こいうシチュエーションも考えられますが、その場合は試合そのものが成立しないってことかな?)。
●There are no substitute players.
サブのメンバーはいません。
●There is no extra time applied.
延長戦もありません。
Observations and Actions(観測と実行)
On each turn, agent receives observations representing full state of the game, including current score, position of all players, their speed, and tired factor. Detailed format of observations is described here:https://github.com/google-research/football/blob/master/gfootball/doc/observation.md#raw-observations
各自順番が来たら、エージェントは、現在のスコア、すべてのプレーヤーの位置、速度、疲労要因など、ゲームの完全な状態を表す観測値を受け取ります。 観測の詳細な形式はここに記述されています:https://github.com/google-research/football/blob/master/gfootball/doc/observation.md#raw-observations
In each turn, agent is supposed to generate one of 19 actions (numbered from 0 to 18) from the default action set:
https://github.com/google-research/football/blob/master/gfootball/doc/observation.md#default-action-set.
その番になったら、エージェントはデフォルトのアクションセットから19のアクション(0から18までの番号)の1つを生成することになっています:
https://github.com/google-research/football/blob/master/gfootball/doc/observation.md#default-action-set。
Returning an action outside of the action set results in agent’s loss.
アクションセット外のアクションを返すと、エージェントが失われます。
Game End(ゲーム終了)
Game ends after 3000 turns or when one of the agents has an error (either timeout, thrown exception, or invalid action returned). The agent who caused an error loses; the other wins. In case of no errors, the winning team is the one which scored more goals. Ranking is then updated according to the evaluation rules. Check out the evaluation tab for more details.
3000回プレイ(1500ステップ x 2)した後、またはエージェントの1つにエラー(タイムアウト、スローされた例外、または無効なアクションが返された)が発生すると、ゲームは終了します。 エラーを引き起こしたエージェントは負けます。 相手の勝ち。 エラーがない場合、勝ったチームはより多くのゴールを獲得したチームです。 その後、評価ルールに従ってランキングが更新されます。 詳細については、評価(Evaluation)タブをご覧ください。
Source Code(ソースコード)
Our game rules are implemented in the following open source code:
https://github.com/Kaggle/kaggle-environments/blob/master/kaggle_environments/envs/football/football.py
私たちのゲームルールは、次のオープンソースコードで実装されています。
https://github.com/Kaggle/kaggle-environments/blob/master/kaggle_environments/envs/football/football.py
JSON schema:
長くなるので、読まなくてもいいそうです(^^)・・・・・省略
Training RL Agents(強化学習エージェントのトレーニング)
Rule-based agent presented in Getting Started section is a good place to start, but things become more interesting with addition of Reinforcement Learning. For a quick example, you can have a look at the GFootball – train SEED RL agent notebook, which shows how to train a V-Trace agent using SEED RL.
「Getting Started」セクションに示されているルールベースのエージェントは、開始するのに適した場所ですが、強化学習を追加すると、状況はさらに興味深いものになります。 簡単な例として、GFootball-train SEED RLエージェントノートブックを見ることができます。これは、SEEDRLを使用してV-Traceエージェントをトレーニングする方法を示しています。
Kaggle Notebook might not be sufficient to train a comprehensive agent for the competition. There are a number of other alternatives worth mentioning:
Kaggle Notebookは、競争のための包括的なエージェントをトレーニングするのに十分ではないかもしれません。 言及する価値のある他の多くの選択肢があります:
●Use GPU-enabled Kaggle Notebooks to speed up the training process. Note that this makes sense when training the agent is more expensive than running the environment.
GPU対応のKaggleNotebookを使用して、トレーニングプロセスをスピードアップします。 これは、エージェントのトレーニングをGoogle環境で実行するよりももっとコストをかける場合に意味があることに注意してください。
●Running training locally on your workstation / server(s). For ways on how to start that, have a look at Google Research Football GitHub repository. You can find instructions on how to train example PPO agent here.
ワークステーションやサーバーでローカルにトレーニングを実行します。 それを開始する方法については、Google Research FootballGitHubリポジトリをご覧ください。 サンプルのPPOエージェントをトレーニングする方法については、ここをご覧ください。
●You can give SEED RL a try. There is a nice blog-post from Warsaw University students who conducted a research project using Google Research Football environment and SEED RL.
SEEDRLを試してみることができます。 Google Researchフットボール環境とSEEDRLを使用して研究プロジェクトを実施したワルシャワ大学の学生からの素晴らしいブログ投稿があります。
●It is possible to train your agent using any other RL framework or implementing your own.
他のRLフレームワークを使用するか、独自のフレームワークを実装して、エージェントをトレーニングすることもできます。
Don’t limit yourself to applying existing tools and methods. Try to invent better ways of training strong agents.
既存のツールや方法を適用することに限定しないでください。 強力なエージェントをトレーニングするためのより良い方法を発明してみてください。
Googleからもらえるボーナスのようなもの・・・・・省略
Appendix
強化学習って何?
Leave a Reply